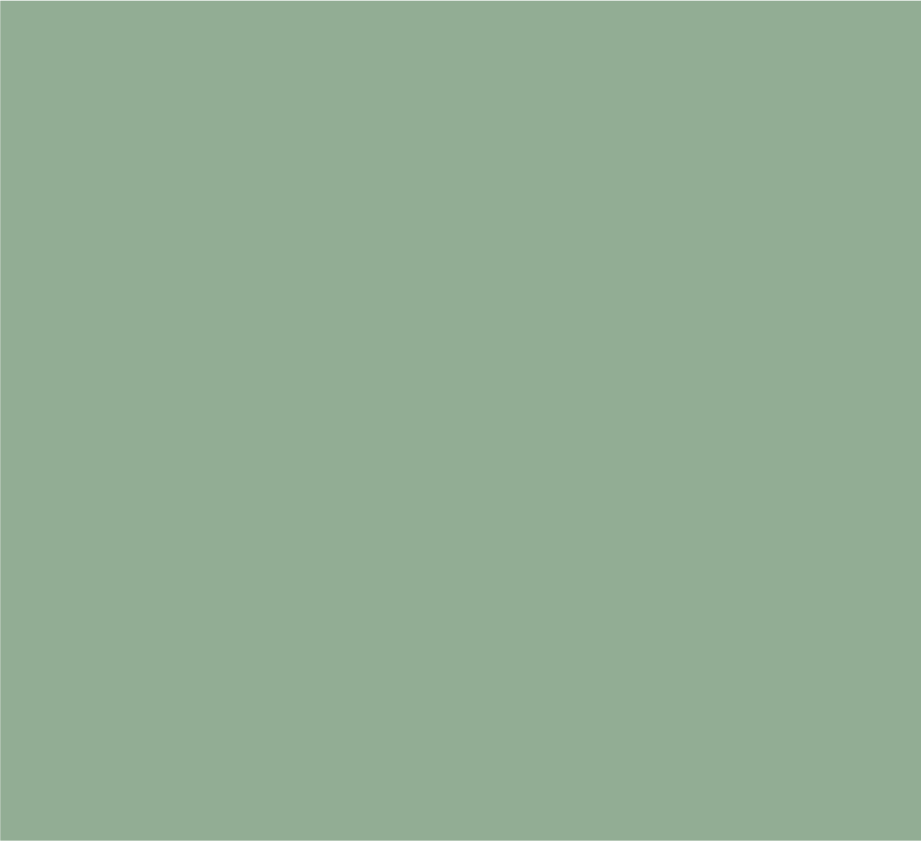
STEP 4: MODEL PROTOTYPING, TUNING, ASSESSMENT
Have all risks been considered?
Risks must be comprehensively mapped and mitigation plans developed in conjunction with the local ecosystem. Potential errors, bias, and discrimination must be considered both at the individual and community level, and viable safeguards identified to prevent harmful consequences for end users / those affected by an AI/ML solution. Since political and technological environments are continuously evolving, risks and contingency plans must be regularly reevaluated and readapted to specific contexts.
Please find below a legend of what can be found within the framework:
📚Resources - e.g. reports, articles, and case studies
🛠Tools - e.g. guidelines, frameworks and scorecards
🔗Links - e.g. online platforms, videos, hubs and databases
❌Gap analysis - tools or resources are currently missing
👥 List of stakeholders which should be included in the specific decision point
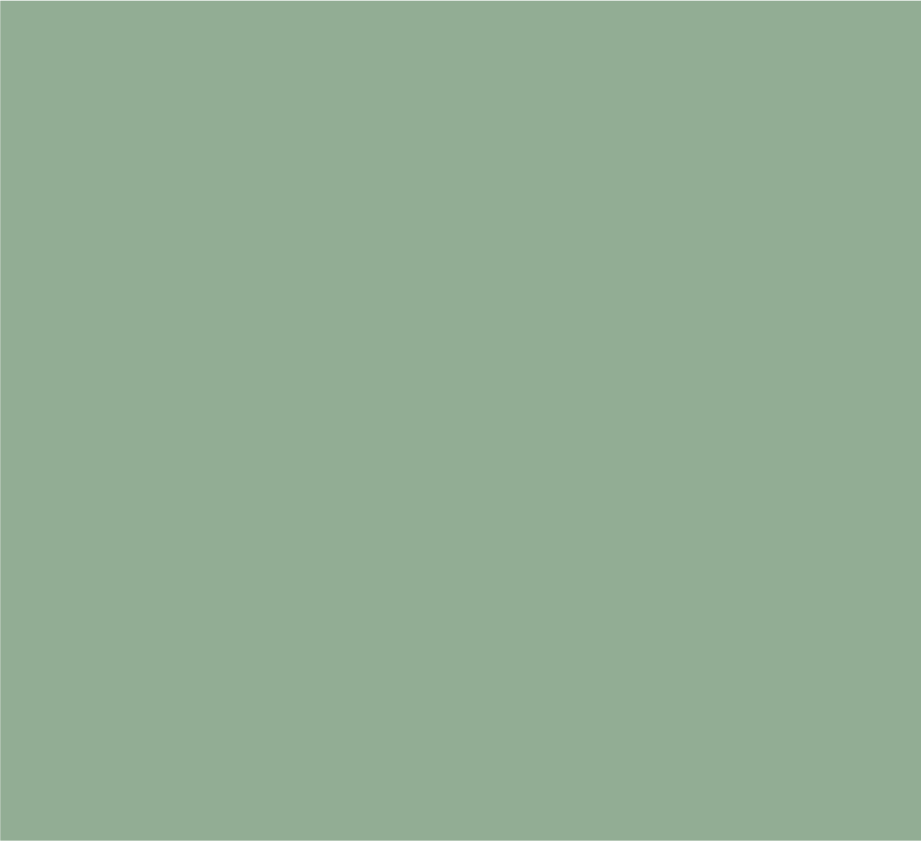
-
👥Civil society, end users, government ministries, technical experts, legal experts, people in the community who might not use / benefit directly from the product but may be indirectly impacted
💭Example list of specific risks to be considered: (1) Discrimination, (2) Inaccuracies, (3) Automation bias, (4) Opacity, (5) Lack of explainability, (6) Lack of accountability, (7) Threats to privacy, (8) Threats to legitimacy and public trust in agencies
🛠 USAID Guide Managing Machine Learning Projects in International Development (page 36 and 39) - List of tools helpful for identifying different types of risks and safeguards when implementing an ML model, and guide to evaluating the level of risk within an ML project, outlining different approaches for lowering risk
🛠 INCASE Framework - A tool to help policymakers and communicators anticipate potential unintended behavioural consequences of a campaign or intervention. It provides useful prompts for consideration early in the design and planning process
🛠 GSMA: AI Ethics Playbook, Self-Assessment Questionnaire (Page 23) - Self-assessment questionnaire that helps establish the risks an AI system may pose and work through steps to mitigate them. To access the questionnaire please contact aiforimpact@gsma.com
📚 Case Study: Afghanistan - Case study on the unintended use of biometric data and on the importance of considering longer term implications and risks of unintended consequences.
❌Tools to perform political economy analysis to assess longer term risks of AI/ML interventions
-
📚Algorithmic Accountability for the Public Sector - This report presents evidence on the use of algorithmic accountability policies in different contexts from the perspective of those implementing these tools, and explores the limits of legal and policy mechanisms in ensuring safe and accountable algorithmic systems