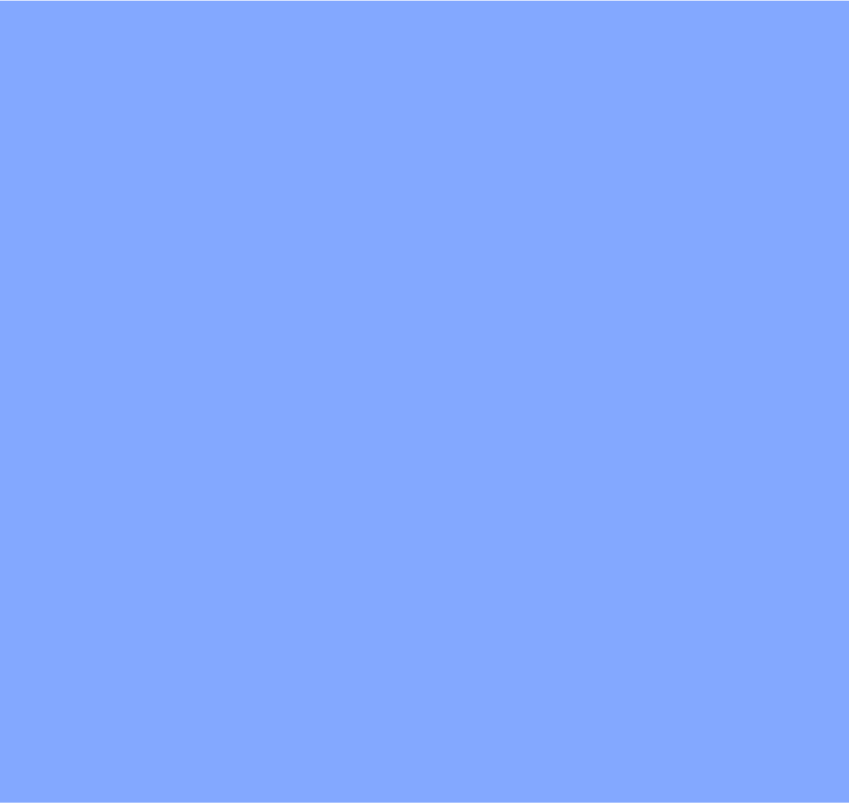
STEP 3: DATA COLLECTION, SELECTION, AND PREPARATION
How is data selected and reviewed and who does it?
During this stage it is crucial to make sure existing data sources have been identified in order to avoid duplication. Once identified, data must be selected and reviewed by a variety of stakeholders, especially those who come into direct contact with the problem. This will highlight what additional data is needed and provides an opportunity to enhance representation. For example, technical developers may be unaware of certain stigmatised groups that are underrepresented or even invisible in a data set, and fail to account for that bias in the training model. migrant communities and internally displaced persons are for example frequently excluded from censuses, population statistics and other data sets.
It is crucial to avoid framing data as something ‘external’ to stakeholders in order to prevent a disconnect between people and data. The collection and review of open-source data is advised where possible, while acknowledging local users’ capacities and limitations in building off it. In data collection and cleanup, clear internal guidelines and external terms of reference should be developed and used to assess for bias including age, gender and/or set population-representative targets, while also acknowledging the potential limitations in the quality of internally generated data.
Please find below a legend of what can be found within the framework:
📚Resources - e.g. reports, articles, and case studies
🛠Tools - e.g. guidelines, frameworks and scorecards
🔗Links - e.g. online platforms, videos, hubs and databases
❌Gap analysis - tools or resources are currently missing
👥 List of stakeholders which should be included in the specific decision point
-
👥 Local owners of data (e.g. governmental admin data), Data scientists, Universities or other research institutions
🔗 DHIS2 - An open source, web-based platform most commonly used as a health management information system (HMIS). The platform boasts data warehousing, visualisation features, and the possibility for data users and policy makers to generate analysis from live data in real-time. It is the world’s largest HMIS platform
🔗 Google Datasets - Google periodically releases data of interest to researchers in a wide range of computer science disciplines
🔗🛠 Facets: Visualisations for ML datasets - Facets contains two robust visualisations to aid in understanding and analysing machine learning datasets
📚A participatory data-centric approach to AI Ethics by Design - Article presenting a participatory, data-centric approach to AI Ethics by Design, particularly relevant to data activities in the early stages of AI/ML development
📚 Feminist Data Collection: Building a Vision of an Inclusive System - Article that examines recommendations and examples of how to implement the principles of data feminism
📚Case study on contextualising collected data (page 3) - Example of how Makerere University’s research projects contextualised collected data on Covid-19 by bringing together diverse perspectives
❌ Tools on how to decide between different types of data storage, tools on assessing data representativeness so as to avoid the widening of the digital divide, data consent practices
-
📚Survey on Bias and Fairness in Machine Learning - Article outlining 23 types of bias in data for machine learning and linked to a more in-depth paper.
📚 Debiasing the Algorithm - This chapter explores the ethics, definitions, and metrics of conversations on fairness, and explores how even when models take these into account, how they are deployed and used in the real world matters just as much
🔗 Responsible AI: From theory to practice (min. 27) - Video exploring data journeys and reflecting on where bias can be introduced